An excellent deseasonalizer is the human brain. Inspecting the unadjusted graph of monthly non-agri employment in the USA below, it is clear at once how the employment situation is developing. Then why do we need programs to seasonally adjust these observations?
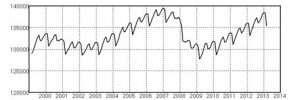
USA non-agri employment
Time series are monitored to keep at current of the most recent developments, as an indication of what to expect in the near future. Then we want to know, in detail, how to evaluate the last observation. That is, we want a picture without the disturbance of seasonal fluctuations. But the leading adjustment procedures give only a ‘provisional’ seasonal adjustment of that recent observation, that will be revised during the following three years. And who is interested, after three years, how the outcome of a monthly or quarterly figure has been revised? It is the present we want to know!
Revisions are caused by the use of centered moving averages: the value of an observation is compared to an average value over a number of full years. In these average values all seasonal ups and downs are present and compensate each other: then comparison with a seasonal observation renders the seasonal fluctuation. However, we can only compare the observation that is then in the middle of the averaged stretch of time. If the average is taken over seven years (the length of a business cycle), than the compared observation must be 3.5 years old.
This is where Camplet comes in. Camplet does not compare values of observations with average values, it compares average change between observations with the change of the recent observation over the previous one. And average change is known up to the very last observation. The seasonal component is updated in order to maintain equality of average change between the data. Consequently Camplet knows no revisions.